Abstract
Orientation: Gaining a full understanding of employee retention (ER) management requires studying multiple retention factors in tandem. Many empirical studies that use conventional structural equation modelling (SEM) include only a single retention factor or a subset of factors, making it impossible to assess the relative embeddedness of these factors in ER practices.
Research purpose: The purpose was to gain a better understanding of the relationships between multiple ER factors and attitudinal antecedents of voluntary turnover.
Motivation for the study: This research aimed to address the need for more comprehensive latent multivariate approaches to studying ER by using extended SEM techniques.
Research approach/design and method: The researchers used a cross-sectional survey design and obtained a convenience sample of 272 skilled employees from public and private organisations. The first stage of the study entailed using the exploratory structural equation model (ESEM) within the confirmatory factor analysis to test a model measuring ER factors. The second stage involved using plausible values for latent variables in an SEM analysis of the relationship between attitudinal antecedents of voluntary turnover (i.e. affective commitment, job satisfaction and turnover intention) and ER factors.
Main findings: The findings indicated that affective commitment and job satisfaction differentially mediated the relationship between ER factors and turnover intention, partially supporting existing research and providing new insights into ER.
Practical/managerial implications: This study suggested that in order for management to effectively manage ER, they must understand the relative embeddedness of a range of ER factors and prioritise motivational and empowerment-enhancing bundles of practice (e.g. compensation, job characteristics, work–life balance and career opportunities) to impact on attitudinal antecedents of voluntary turnover.
Contribution/value-add: This study indicated that the use of extended SEM modelling techniques could provide valuable insights into the multivariate relationships between ER factors and attitudinal antecedents of voluntary turnover.
Keywords: affective commitment; employee retention; exploratory structural equation modelling; job satisfaction; turnover intention.
Introduction
Maintaining a productive workforce and retaining existing employees are central issues in the competitive, dynamic and volatile world of business (Gani, Potgieter, & Coetzee, 2020). Employee retention (ER) factors (e.g. compensation, training and development, promotion opportunities, work–life balance, managerial support and job design) play a key role in retaining employees and preventing them from searching for greener pastures (Al-Emadi, Schwabenland, & Wei, 2015; Das & Baruah, 2013; Lee, Hom, Eberly, & Li, 2018). According to Ghosh, Satyawadi, Prasad Joshi and Shadman (2013), the retention strategies of organisations should incorporate multiple ER factors.
De Vos and Meganck (2009), Guchait and Cho (2010) and Subramony (2009) point out that studying multiple ER factors in tandem is necessary to gain a full understanding of retention management. Most empirical studies address only one retention factor or a subset of factors, making it impossible to assess the embeddedness of these factors in ER practices (Al-Emadi et al., 2015; De Vos & Meganck, 2009). Following a multifaceted approach (i.e. considering multiple ER factors) to ER management as opposed to one that focuses on single ER factors produces significantly superior outcomes (Dechawatanapaisal, 2018). This highlights the need for more comprehensive multivariate approaches to studying ER (Hom, Lee, Shaw, & Hausknecht, 2017; Lee et al., 2018). Existing research has identified many ER factors (Rubenstein, Eberly, Lee, & Mitchell, 2015, 2017), but our study focused on the most common and critical ER factors associated with human resource management (HRM) practices, namely, compensation, job characteristics, training and development opportunities, supervisor support, career opportunities (CO) and work–life balance (Döckel, 2003; Döckel, Basson, & Coetzee, 2006; Gani et al., 2020; Potgieter & Snyman, 2018).
Although numerous studies connect ER practices and job satisfaction, affective commitment and turnover intention (Lee et al., 2018; Rubenstein et al., 2015, 2017), multivariate studies on the simultaneous relationships between multiple ER practices and these attitudinal antecedents of voluntary turnover intention are scarce (Al-Emadi et al., 2015; Dechawatanapaisal, 2018; Guchait & Cho, 2010; Juhdi, Pa’wan, & Hansaram, 2013; Subramony, 2009). Existing literature suggests that employee turnover intention, job satisfaction and affective commitment are important proximal antecedents to voluntary turnover and also significant descendants of more distal ER factors (Duarte, Gomes, & Neves, 2015; Lee et al., 2018; Rubenstein et al., 2015, 2017). Therefore, an extended SEM analysis that includes all these constructs should contribute significantly to an understanding of the multivariate nature of ER practices in organisations.
This study specifically addressed the need for analysing multiple ER variables and attitudinal antecedents of voluntary turnover (turnover intention, job satisfaction, affective commitment) in tandem. To do this, we used an expanded measurement model and latent multivariate analysis techniques. Using extended techniques within the structural equation modelling (SEM) framework, we analysed complex relationships between multiple constructs of sufficient breadth and depth to ensure valid and accurate findings. Structural equation modelling is considered the preferred method for conducting multivariate analyses with latent variables that control for measurement error (Devlieger & Rosseel, 2017). SEM estimates all parameters in a measurement model and a structural model simultaneously, thereby eliminating bias in path coefficients (Devlieger & Rosseel, 2017). However, conventional confirmatory factor analysis (CFA) identifies all cross-factor item loadings as model misspecifications and can be considered too restrictive when testing lengthy measurement models and imperfect indicators typically found in the social sciences (Marsh, Morin, Parker, & Kaur, 2014). This may explain why previous studies incorporated limited ER factors in SEM models. Shortening measures, using item parcels and aggregating factor scores are popular strategies commonly employed to overcome model misspecification challenges and obtain good fitting SEM models. However, these strategies erode the depth and breadth of the measured constructs, discount measurement error and camouflage misspecification in CFAs and SEMs (Hsu, Skidmore, Li, & Thompson, 2014; Marsh et al., 2009). To overcome these challenges, exploratory structural equation modelling (ESEM) and plausible values (PVs) can be used.
Compared to CFA, ESEM is more flexible as it accommodates multiple and minor cross-factor item loadings and provides a more realistic representation of lengthy measurement models that typically contain imperfect indicators (Marsh et al., 2014). Moreover, PVs (i.e. latent scores) that accurately represent the latent variables of a measurement model can effectively be used in an SEM structural model without having to include the full measurement model (e.g. the ESEM or CFA model). Plausible values account for measurement error, have less computational demands and counter model misspecification issues and sample-size demands (Asparouhov & Muthén, 2010). In the present study, ESEM and PVs are regarded as extended CFA techniques. More details on these techniques are presented in the ‘Analytical approach and statistical analyses’ section. Mai, Zhang and Wen (2018) confirm that using ESEM models instead of the conventional CFA models in SEM analyses results in less biased path coefficients where minor, but non-ignorable, cross-factor item loadings exist.
Research purpose
The purpose of this study was to gain a comprehensive understanding of the simultaneous effect of multiple ER factors on attitudinal antecedents of voluntary turnover (affective commitment, job satisfaction and turnover intention) through the testing of a multivariate SEM model and the use of extended SEM techniques.
Similar to recent studies published in high-impact management and psychology journals (Howard, Gagné, Morin, & Forest, 2018; Sánchez-Oliva et al., 2017), our study followed a ‘methodological–substantive synergy’ approach (Marsh & Hau, 2007, p. 152), which entails applying methodological developments and innovative statistical tools with enhanced precision to complex substantive issues, resulting in advancing theory.
In the ‘Literature review’ section, we discuss the theory that informed this study’s hypotheses relating to the relationship between ER factors and the attitudinal antecedents of voluntary turnover. For purposes of conciseness, we relied strongly on a synthesis of findings drawn from the existing seminal works, review studies and meta-analyses to support our study’s hypotheses.
Literature review
Employee retention
Retaining employees is a significant factor in organisations’ talent management strategies to reduce turnover costs (Narayanan, Rajithakumar, & Menon, 2019). For HRM policies and practices to be effective, they should take account of the ER factors. This study supports Gani et al.’s (2020, p. 144) definition of retention factors: ‘Retention practices are those organisational context factors that influence an employee’s choice to continue to work for an organisation or to leave the organisation’.
Although a variety of theories relating to social identity, work motivation, employee equity and expectancy contribute to explaining ER (Hom et al., 2017), the social exchange theory has been widely used more than others to gain an understanding of social behaviour and interactions (Al-Emadi et al., 2015). An important finding has been that employers’ actions in respect of valued retention factors reinforce employees’ intention to stay or leave (Al-Emadi et al., 2015).
Next, we present a brief discussion of each of the attitudinal antecedents of voluntary turnover (turnover intention, job satisfaction, affective commitment) and the ER factors included in this study, and we refer to our hypotheses statements.
Turnover intention
Turnover intention is defined as ‘a conscious and deliberate wilfulness to leave the organization’ (Matz, Woo, & Kim, 2014, p. 234). Compared to other turnover antecedents (e.g. job satisfaction, affective commitment), turnover intention is the most significant predictor of actual turnover (typically sharing 25% of the turnover variance; Rubenstein et al., 2017). Researchers suggest that job satisfaction and organisational commitment are linked and are both essential attitudinal determinants of turnover intention (Al-Emadi et al., 2015; Joseph, Ng, Koh, & Ang, 2007; Kim & Kao, 2014; Larkin, Brantley-Dias, & Lokey-Vega, 2016; Luna-Arocas & Camps, 2007; Nouri & Parker, 2020; Price & Mueller, 1981; Tett & Meyer, 1993).
Job satisfaction
Job satisfaction manifests in employees’ feelings about their jobs, which are the result of subjective evaluations of job characteristics (Agho, Price, & Mueller, 1992). Job satisfaction may indicate positive feelings about specific aspects of a job or about a job in general. This study defines job satisfaction as a positive feeling about a job in general—a feeling that (Weiss, Dawis, England, & Lofquist, as cited in Larkin et al., 2016):
[D]epends on how closely a person’s abilities match the requirements of the job and the extent to which a person’s needs are fulfilled by reinforcing aspects of the work environment. (p. 28)
The negative relationship between job satisfaction and turnover intention has been well-documented (Guzeller & Celiker, 2019).
Organisational commitment
According to Allen and Meyer (1990), organisational commitment refers to an employee’s wish to stay with an organisation because of its characteristics, goals and values. These authors identify three facets of organisational commitment: affective commitment or emotional attachment, continued commitment that takes the cost of leaving the job into consideration and normative commitment that considers contractual obligations to the organisation. The focus of the present study is on affective commitment. Research evidence suggests that the relationship between turnover intention and affective commitment overshadows the relationship between turnover intention and the other forms of commitment (Allen & Meyer, 1990). As such, the former relationship determines employees’ attitudes to turnover intention and retention (Meyer & Herscovitch, 2001; Nouri & Parker, 2020; Solinger, Van Olffen, & Roe, 2008).
The literature provides evidence relating to the directional relationships between ER factors and attitudinal determinants of voluntary turnover, and we deal with this aspect next.
Direct relationships between employee retention factors and attitudinal antecedents of voluntary turnover
In our discussion of the research on the direct relationship between ER factors and the attitudinal antecedents of voluntary turnover (i.e. turnover intention, job satisfaction and affective commitment), we refer to the measurement model of Döckel (2003) which includes the constructs of compensation, job characteristics, training and development opportunities, supervisor support, CO and work–life balance. Döckel’s (2003) measurement model is based on well-known and often-cited ER factor measures developed, validated and cross-validated by other researchers in the field. In our discussion of meta-analyses studies, we also included the mean effect sizes ( ) (Cohen, 1987) reported, because of their high generalisability.
Compensation
Compensation (C) includes monetary and non-monetary rewards offered to employees in return for services rendered (Ibidunni, Osibanjo, Adeniji, Salau, & Falola, 2016). In Döckel’s (2003) measurement model, compensation incorporates content from Heneman and Schwab’s (1985) measure that focuses on the importance of employees’ satisfaction with compensation, benefits, raises, and the structure and administration of remuneration systems. Moncarz, Zhao and Kay (2009) and Singh (2019) argue that compensation can be a critical factor in reducing turnover and increasing commitment and also in motivating employees to leave organisations. Meta-analysis studies in a variety of work environments show compensation has a large effect ( = 0.45) on affective commitment, a small to medium effect ( = –0.23; = –0.37) on job satisfaction and a medium to large direct effect ( = –0.42; = –0.60) on turnover intention (Joseph et al., 2007; Kim & Kao, 2014; Meyer, Stanley, Herscovitch, & Topolnytsky, 2002; Saber, 2014). However, the effect sizes reported varied considerably from study to study, suggesting the context of a study may be a determining factor.
Job characteristics
McKnight, Phillips and Hardgrave (2009) define the construct of job characteristics (J) as employees’ perceptions regarding the nature and content of a task. The measurement model of Döckel (2003) includes the job characteristics of skill variety and autonomy, which were derived from Hackman and Oldham’s (1976) job diagnostic survey. If work is autonomous and challenging, offers variety and requires superior skills, employees also develop feelings of job satisfaction, commitment and intention to stay (Bontis, Richards, & Serenko, 2011; Döckel, 2003). Meta-analysis studies show that job characteristics have a medium to large effect ( = 0.38; = 0.50) on job satisfaction (Saber, 2014) and a small to large effect ( = −0.22; = −0.50) on turnover intention (Matz et al., 2014). Thus, evidence suggests that job characteristics should have a medium to large effect on the antecedents of voluntary turnover.
Training and development opportunities
Through the formal training and development activities (T) an organisation provides, employees obtain the information they need to fulfil their job duties (Bernhard & O’Driscoll, 2011). Döckel (2003) bases the general professional development opportunities and the resourcing and availability of relevant job- and product-specific training opportunities in his measurement model on the training items developed by Rogg, Schmidt, Shull and Schmitt (2001).
Employees whose organisations give them opportunities for learning and growing will continue to work for their organisations and will be less inclined to leave (Ghosh et al., 2013). Ready availability of training and career development opportunities also result in increased job satisfaction (Deery, 2008; Ibidunni et al., 2016). According to Aladwan, Bhanugopan and D’Netto (2015) and Gani et al. (2020), training and development have a positive effect on organisational commitment. However, Chew and Chan (2008) have found that although training and development opportunities correlate positively with the intention to stay, they do not show a significant correlation with affective commitment. Findings about the relationship between training and development and organisational commitment appear to be inconsistent (Smeenk, Eisinga, Teelken, & Doorewaard, 2006).
Supervisor support
Supervisor support (S) consists of the recognition and feedback employees receive from supervisors (Döckel et al., 2006). Döckel’s (2003) model includes several items that Ramus and Steger (2000) developed to measure supervisor recognition as well as items that Good and Fairhurst (1999) developed to measure supervisor feedback. Supervisors’ feedback and recognition increase employees’ self-worth and affective commitment and may even prevent them from leaving the organisation or being absent from work (Van Dyk & Coetzee, 2012). Relationships with supervisors and other colleagues can influence employees’ satisfaction and their decision to leave or not leave the organisation (Ibidunni et al., 2016). Meta-analysis studies have found supervisor support to have a medium effect ( = −0.32) on turnover intention (Kim & Kao, 2014; Matz et al., 2014). Overall, the literature suggests significant relationships between supervisor support and the antecedents of voluntary turnover (Rathi & Lee, 2017).
Career opportunities
Based on items that Landau and Hammer (1986) developed, Döckel (2003) describes CO as including internal promotions or lateral moves to different positions in an organisation. In their study, Kochanski and Ledford (2001) found that the reward of being offered career development opportunities was the most prevalent retention factor. Research by Gani et al. (2020), Joāo and Coetzee (2012) and Kraimer, Seibert, Wayne and Liden (2011) shows that perceived career development opportunities and career growth have a significant positive impact on employees’ affective commitment and job performance and reduce their intention to leave the organisation. Promotions, career development opportunities and appreciation in the workplace have a great impact on employees’ job satisfaction (Ibidunni et al., 2016; Sheraz, Batool, & Adnan, 2019).
Work–life balance
Kraimer et al. (2011) define work–life balance (W) as employees’ ability to meet work and family commitments as well as other non-work responsibilities and activities. Döckel’s (2003) model includes work–life balance items from Paré, Tremblay and Lalonde’s (2001) measure and focuses on the extent to which the demands of a job and a work–life conflict with the demands of a family and a personal life. Richman, Civian, Shannon, Jeffrey Hill and Brennan (2008) associate perceived flexible working arrangements and the ability to balance the demands of a personal and a work life with increased levels of employee engagement, higher levels of productivity and job satisfaction, and a decrease in turnover intention. In a longitudinal study, Nohe and Sonntag (2014) found that work–family conflict had a medium effect (r = 0.36) on turnover intention. Similar findings were made by Chan and Ao (2019) in their cross-sectional study. Meta-analysis studies indicate that work–family conflict has a medium effect ( = −0.25; = −0.26) on job satisfaction (Butts, Casper, & Yang, 2013; Shockley & Singla, 2011) and a small effect ( = −0.17) on affective commitment (Butts et al., 2013).
Having reviewed existing research on the direct relationship between ER factors and the antecedents of voluntary turnover, we formulated Hypothesis 1:
Hypothesis 1: ER factors show a statistically significant (p < 0.05) direct positive effect on affective commitment and job satisfaction and a statistically significant (p < 0.05) direct negative effect on turnover intention.
Relevant to our study was the relationships amongst attitudinal antecedents of voluntary turnover, and we reviewed applicable research in this regard.
The relationships amongst attitudinal antecedents of voluntary turnover
Researchers identify job satisfaction as an antecedent to organisational commitment, and organisational commitment as a mediator of the relationship between job satisfaction and turnover intention (Farrell & Rusbult, 1981). However, research findings differ as to the direction and nature of the relationship between job satisfaction and organisational commitment (Farkas & Tetrick, 1989). These findings include models indicating organisational commitment to causally affect job satisfaction, and models indicating a reciprocal relationship between job satisfaction and organisational commitment (Farkas & Tetrick, 1989). More recent studies on ER tend to adopt models with paths running from job satisfaction to organisational commitment (Al-Emadi et al., 2015; Ćulibrk, Delić, Mitrović, & Ćulibrk, 2018; Valaei & Rezaei, 2016). Both organisational commitment and job satisfaction have been shown to contribute uniquely to turnover intention (Tett & Meyer, 1993), suggesting that organisational commitment may not fully mediate the path between job satisfaction and turnover intention.
The aforegoing theoretical discussion supports our study’s second hypothesis:
Hypothesis 2: Job satisfaction has a significant indirect and significant direct negative effect (p < 0.05) (i.e. partial mediation) on turnover intention via the mediator of affective commitment.
As the direct and indirect relationships between ER factors and attitudinal antecedents of voluntary turnover were relevant to our study, we reviewed the applicable literature in this regard.
Direct and indirect relationships between employee retention factors and attitudinal antecedents of voluntary turnover
Research suggests that ER factors may be distal determinants of turnover intention and that their effects are mediated by job satisfaction and affective commitment as proximal attitudinal determinants of turnover intention (Duarte et al., 2015; Hom, Mitchell, Lee, & Griffith, 2012; Joseph et al., 2007; Tett & Meyer, 1993). Exchange theory – an expansion of the interdependency theory (Homans, 1961; Kelley & Thibaut, 1978) – supports the direction of the paths of a model according to which job satisfaction depends on the specific and immediate rewards and costs related to the job (e.g. job characteristics). Furthermore, affective commitment to the organisation – a broader concept with a longer-term perspective – depends on job satisfaction and other rewards and costs related to the organisation in general (e.g. compensation and benefits; Farrell & Rusbult, 1981). We hypothesised an unfolding model where ER factors indirectly affected the turnover intention via job satisfaction and affective commitment, and we assumed that affective commitment partially mediated the path between job satisfaction and turnover intention.
The above theoretical discussion supports the study’s third hypothesis:
Hypothesis 3: ER factors show a statistically significant (p < 0.05) indirect negative effect and a statistically insignificant direct effect (i.e. full mediation) on turnover intention via the mediators of job satisfaction and affective commitment.
Our study’s purpose was to gain a more comprehensive understanding of the effect of multiple ER factors on the attitudinal antecedents of voluntary turnover (affective commitment, job satisfaction and turnover intention) through the testing of a multivariate SEM model and the use of extended SEM techniques.
Research design
Research approach
We used a quantitative approach and a cross-sectional survey design to study the relationships between ER factors and the attitudinal antecedents of voluntary turnover.
Research participants
A convenience sample of 272 skilled, highly skilled or professional employees from various public and private organisations in South Africa was obtained. The response rate was 47%. The majority of the sample consisted of white people (65%), English speaking (78%) and women (64%). These participants had obtained a tertiary qualification (76%) and had been employed in their respective companies for a period of less than 5 years (64%).
Measuring instruments
We used a number of instruments, each based on a Likert-type scale. To measure the distal determinants of attitudinal antecedents of voluntary turnover, we used the Retention Factor Scale (RFS; Döckel, 003) consisting of the subscales of compensation satisfaction (C), job characteristics (J), training and development (T), supervisor support (S), career opportunities (CO) and work–life balance (W). As measures of attitudinal antecedents of voluntary turnover, we used the Job Satisfaction Scale (JSS; Hellgren, Sjöberg, & Sverke, 1997), the Affective Commitment Scale (ACS; Meyer & Allen, 1991) and the Turnover Intention Scale (TIS; Sjöberg & Sverke, 1996). A biographical questionnaire was administered to the respondents. The measures we used (for a detailed summary, see Table 1) are well known and have been extensively used and frequently cited (Döckel et al., 2006).
TABLE 1: Summary of measurement instruments used. |
Analytical approach and statistical analysis
This study used two extended CFA techniques to overcome the limitations of the overly restrictive conventional CFA model, which penalises for trivial non-target cross loadings. Firstly, ESEM, nested in CFA (EwC), was used to test the RFS being a complex and multidimensional model. Secondly, PVs were used as factor scores to accurately represent the latent variables of the RFS model, which obviated the need to include the full measurement model in the SEM analysis. We used the MPlus Statistical Software Version 7.3 and the maximum likelihood (ML) estimation method with robust standard errors (MLR) to conduct the SEM analyses (Muthén & Muthén, 2012). We relied on the full-information MLR estimator to adequately account for missing data (Muthén & Muthén, 2012). Overall, 2.1% of the data out of the total dataset was missing at random.
We conducted ESEM and target rotation as precursors to the EwC analyses. Exploratory structural equation modelling, which integrates conventional CFA and exploratory factor analysis (EFA), does not penalise for trivial non-target cross loadings. Our decision to set to zero all item loadings not expected to relate to a latent variable was based on theoretical and empirical grounds. We used the EFA solution of Döckel (2003, p. 160) to set the target loadings. The results of Döckel’s EFAs of the RFS show small-item cross loadings with orthogonal rotation and significant inter-correlations between variables.
Asparouhov and Muthén (2009) suggest that, in the case of a study (such as ours) that uses a relatively small sample, conducting an EwC nested in an ESEM and fixing all statistically insignificant cross loadings to zero, reduces the number of parameters that need to be estimated and results in more precise estimates. We used the EwC model as the measurement model in the SEM analysis to determine the relationships between RFS and the criterion variables (Crt) of ACS, JSS and TIS. For the EwC, we applied the procedure of Morin, Marsh and Nagengast (2013) in using all parameter estimates in the ESEM solution as starting values and, except for placing m2 restrictions (m = number of factors), freely estimating them in the EwC model. After confirming that all parameter estimates and fit indices’ values of the EwC model replicated those of the ESEM model, we restricted all insignificant cross-loading estimates for factor indicators to ‘0’ as proposed by Asparouhov and Muthén (2009) and tested the restricted EwC model depicted in Figure 1.
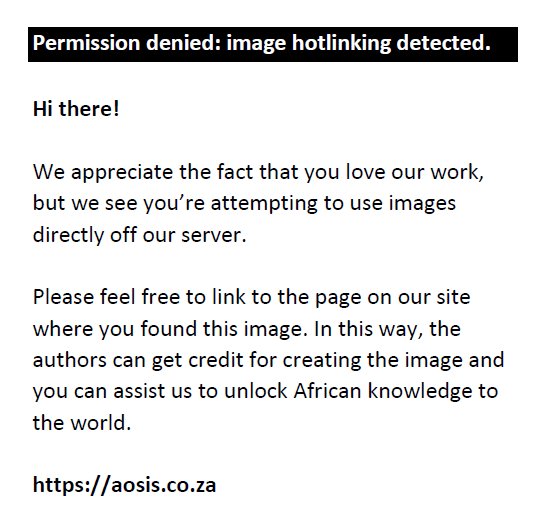 |
FIGURE 1: The restricted exploratory within confirmatory (EwC) structural equation measurement model tested for the Retention Factor Scale. |
|
In determining the quality of the RFS measurement model, given sample size (N = 272; Muthén & Muthén, 2002), we performed a Monte Carlo (MC) simulation analysis using MPlus. We used 10 000 random sample estimates to determine the 95% coverage and statistical power of the model estimates (> 0.80), parameter estimate and standard error bias (< 5%).
Using CFA, we tested the discriminate and structural validity of the measures of attitudinal antecedents of voluntary turnover (ACS, JSS and TIS), also referred to as the Crt measurement model.
To test Hypotheses 1, 2 and 3, we used PVs for W, CO, S, T, J and C instead of including the full RFS measurement model in the SEM structural model (Figure 2) because such use in a secondary SEM analysis imposes fewer computational and sample-size demands. Figure 2 depicts the SEM path model for the PVs of the RFS (EwC model) on the attitudinal antecedents of voluntary turnover (Crt model), hereafter referred to as EwC-on-Crt1&2. Only the black regression paths in Figure 2 (and not the red regression paths running between the latent variables JSS, ACS and TIS) reflect Crt1 model, whereas the black and red regression paths reflect the Crt2 model. We used the MLR estimator to test the EwC-on-Crt1&2 models, which includes both the Crt1 and the Crt2 models. Using the delta method, we estimated the standard errors and calculated the statistical significance of indirect paths or effects. According to Muthén and Muthén (2012), the MLR estimation procedure calculates the standard error estimates that are robust to non-normal data and is considered similar to the bootstrap technique for estimating standard errors.
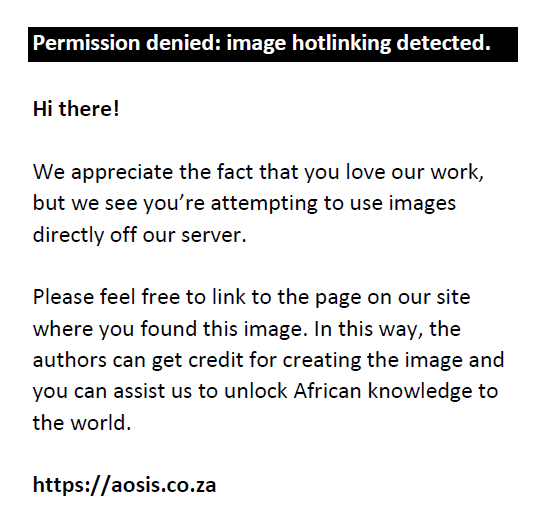 |
FIGURE 2: The structural equation path model (EwC-on-Crt1&2) of the relationship between the variables of the Retention Factor Scale and the attitudinal antecedents of voluntary turnover scales. |
|
In estimating the PV data sets, we used multiple imputation procedures (which are commonly used for handling missing data) and the Bayes estimator (Asparouhov & Muthén, 2010). To avoid biased parameter estimates, we included in the multiple imputation model all the items and latent variables used in the EwC and Crt models. (see Asparouhov and Muthén [2010, p. 2] for details on the imputation method they call ‘H0 imputation’.) More specifically, the imputation model included the RFS parameters fixed to the values obtained in the EwC model, and, using starter values from the CFA model, we specified the Crt model parameters to be freely estimated. Following the recommendations of Lüdtke, Robitzsch and Trautwein (2018), we imputed 30 PV data sets using MPlus Markov chain MC Bayesian estimation utilities with Gibbs sampler (PX1) and 100 000 iterations (Muthén & Muthén, 2012). We monitored convergence (which should be below 1.05) with the potential scale reduction indicator and trace plots. For all estimates, we adopted the MPlus default settings for diffuse priors, and we combined the data sets using Rubin’s (1987) method.
In the SEM models (EwC-on-Crt1&2) that we used to test Hypotheses 1, 2 and 3, we cannot strictly claim direct or indirect causal effects (i.e. the directionality of model paths) as we used cross-sectional data in the analysis conducted. However, Kline (2015) contends that directionality assumptions for model paths may be assumed if the rationale and supporting knowledge are strong. In this study, we relied strongly on exchange theory principles, seminal works, review studies, empirical and meta-analytic research evidence to support directionality assumptions and inferences in the SEM model. We relied on covariance statistics to support or rule out the probability of direct or indirect causal effects whilst controlling for multiple covariates.
Ethical consideration
With the assistance of the human resource management departments of their respective companies, the respondents completed the surveys in either a paper-based or an electronic format. Participation in the study was voluntary, anonymous and confidential. The relevant ethics committee of the University of Pretoria where the study was conducted approved the use of the data for research purposes (reference no. Nr EMS090/18).
Results
The study’s descriptive statistics showed that the average item or indicator skewness was −0.39 and varied between −1.01 and 0.57. The average kurtosis was −0.61 and varied between −1.24 and 0.89. The data signified a good approximation of the normal distribution (skewness and kurtosis b etween −1 and +1), which can produce robust ML parameter estimates with less dependency on the effect of sample size (Anderson & Gerbing, 1988). All SEM models in the study converged adequately and did not produce improper estimates (e.g. negative residual variances).
Next, we present the results of the study’s statistical analyses relating to the RFS measurement model, the attitudinal antecedents of the voluntary turnover measurement model and Hypotheses 1, 2 and 3.
Model fit was estimated based on several fit indices. Tucker–Lewis index (TLI) and comparative fit index (CFI) values greater than 0.90 and 0.95 typically reflect acceptable and excellent fit to the data (Marsh, Hau, & Wen, 2004). Root mean square error of approximation (RMSEA) and standardised root mean square residual (SRMR) values of less than 0.05 and 0.08, respectively, reflect a close fit and a reasonable fit to the data (Marsh et al., 2004). The Chi-square statistics is sample-size sensitive and presents a risk of a type II error; therefore, we did not use it to evaluate the overall model fit. As expected, all parameter estimates and fit indices of the unrestricted EwC model closely replicated the ESEM model and showed an overall acceptable fit (Table 2). The EwC analyses of the restricted model (all statistically insignificant cross-loadings fixed at zero) showed an acceptable to good model fit (RMSEA = 0.047, CFI = 0.934, TLI = 0.924, SRMR = 0.051; Table 2). The residuals of items Q11 and Q8 were allowed to correlate because they were very similar in wording and highly correlated (r = 0.83), signifying a method artefact.
TABLE 2: The model fit indices for the structural equation models tested in the study. |
The structural loadings of the RFS for the EwC model are presented in Table 3.
TABLE 3: The item loadings on the Retention Factor Scale factors for the restricted exploratory structural equation modelling within the confirmatory factor analysis model and the item loadings on factors for the attitudinal antecedents of the voluntary turnover model (criterion). |
See Table 4 for the inter-correlation coefficients between constructs.
TABLE 4: Inter-correlation matrix of all variables included in the structural equation path model (EwC-on-Crt). |
The EwC model represented a simple factor structure and was well defined with salient loadings (λ > 0.30) on target factors and overall minor cross loadings (λ < 0.30; Table 3) (Thurstone, 1947) except for one item from T that loaded on both T (λ = 0.44) and CO (λ = 0.44). The item (i.e. ‘There are enough development opportunities for me in the company’) may conceptually equally relate to both T and CO. The overall model fit and the factor structure support the RFS measurement model.
The MC analyses for normally distributed data showed that all the parameter estimates of the RFS measurement model had a sufficient 95% coverage rate (i.e. interval = 0.92% – 0.96%), adequate statistical power (i.e. range = 0.36–1.0; mean = 0.96), non-biased parameter estimates (i.e. range = 0% – 5.2%; mean = 0.56%) and non-biased standard error estimates (i.e. range = 0% – 2.61%; mean = 0.94%). Only trivial cross loadings smaller than 0.16 showed inadequate power (i.e. 0.36). According to Gorsuch (1983), cross loadings up to ± 0.10 are generally considered random variations from 0. Overall, we concluded that the measurement model of the RFS showed adequate fit for the sample, was stable and accurate and should form a good basis for latent variable SEM analyses.
Table 3 depicts that the structural parameter loadings of the three-Crt variables (JSS, ACS and TIS) of the Crt measurement model showed an acceptable fit (RMSEA = 0.082, CFI = 0.955, TLI = 0.940, SRMR = 0.036; refer to Table 2). We tested two alternative models: a one-factor model that included all-Crt variable items and a two-factor model where all the ACS and JSS items formed a single factor and the TIS items formed the second factor. Each alternative model demonstrated a relatively much weaker model fit (one-factor model: χ2(44) = 331.187*, RMSEA = 0.162, CFI = 0.805, TLI = 0.756, SRMR = 0.09; two-factor model: χ2(43) = 192.738*, RMSEA = 0.118, CFI = 0.898, TLI = 0.870, SRMR = 0.07). These results showed that the data adequately supported the more parsimonious three-factor model and illustrated the distinctiveness of the three constructs in the context of the study.
Table 4 depicts the pairwise correlation matrix for all RFS PVs and Crt latent variables included in the EwC-on-Crt1&2 structural models (Figure 2). The correlations between RFS factors varied between r = 0.23 and r = 0.59, signifying well-differentiated constructs. The omega factor reliability coefficients varied between 0.63 and 0.95, meeting the minimum level of acceptability (>0.60) (McDonald, 1999). The inter-correlations between the Crt latent variables were high, ranging between r = 0.70 and r = 0.77. However, these values did not support the likelihood of adverse multicollinearity because the highest latent variable inter-correlation (ACS and JSS r = 0.77) was below 0.80 and the omega reliability coefficients were above 0.80 (Grewal, Cote, & Baumgartner, 2004).
In the analysis relating to Hypothesis 1 (The RFS latent variables [PVs] show statistically significant [p < 0.05] positive direct effects on JSS and ACS and statistically significant negative effects on TIS), the ACS, JSS and TIS latent variables (excluding the regression paths connecting them [Figure 2 and rows 1, 2 and 3 of Table 5] of EwC-on-Crt1 were allowed to covary.
TABLE 5: The regression coefficients in the structural equation path model (EwC-on-Crt1&2) Inter-correlation matrix of all variables included in the structural equation path model (EwC-on-Crt). |
The EwC-on-Crt1&2 analysis results are shown in Tables 2 and 5. The EwC-on-Crt1&2 structural models showed an acceptable model fit: RMSEA = 0.057, CFI = 0.964, TLI = 0.951, SRMR = 0.035 (Table 2). The first three rows of Table 5 indicate parameter estimates that apply to Hypothesis 1 and the EwC-on-Crt1 model, but not the regression paths connecting TIS1, JSS1 and ACS1 (see red lines in Figure 2; and only standardised parameter estimates are reported in Table 5). The results showed that the RFS variables had a statistically significant total direct effect when we regressed all the variables on each of the Crt variables. The RFS explained 59%, 61% and 44% of the variance on ACS1, JSS1 and TIS1, respectively, providing strong support for Hypothesis 1. When regressed on ACS1, variables C (β1 = 0.47), J (β2 = 0.29, p < 0.05) and W (β6 = 0.11, p < 0.05) were statistically significantly shown as unique contributors. Variables C (β1 = 0.34, p < 0.05), J (β2 = 0.44, p < 0.05) and W (β6 = 0.23, p < 0.05) were statistically significantly shown as unique contributors when regressed on JSS. The contributions of variables T, S and CO to the regression equation were statistically non-significant in respect of both ACS1 and JSS1 and did not support Hypothesis 1. Variables C (β1 = −0.31, p < 0.05), J (β2 = −0.18, p < 0.05), CO (β5 = −0.26, p < 0.05) and W (β6 = −0.24, p < 0.05) were shown statistically significantly as unique contributors when regressed on TIS1. Variables T and S were statistically non-significant when regressed on TIS1 and did not support Hypothesis 1.
Although the study supported Hypothesis 1 in terms of variables C, J and W, not all the RFS variables (CO, T and S) related directly at a statistically significant level to each of the Crt variables in the structural model or SEM.
For the analysis relating to Hypothesis 2 (Job satisfaction has a statistically significant indirect and direct negative effect [p<.05] [i.e. partial mediation] on turnover intention via mediator affective commitment), we included in the model all the relevant paths between RFS and Crt (Figure 2) as well as the regression paths connecting TIS, JSS and ACS.
The estimated regression paths showed that the latent variable JSS (β7 = −0.27, p < 0.05) had a statistically significant negative direct effect on TIS2 as a unique contributor to the regression model (Table 5, row 5). Also, the indirect effect of JSS on TIS2ACS via ACS was statistically significant (β7 = −0.27, p < 0.05; Table 5, row 6). Therefore, the results supported Hypothesis 2. More specifically, the results suggested a partial mediating effect of ACS on the relationship between JSS and TIS2. Furthermore, the estimated regression paths showed that the mediator variable ACS (β8 = −0.56, p < 0.05) had statistically significant negative direct effects on TIS2. After having controlled for all the RFS covariates in the model, we concluded that both ACS and JSS made a unique contribution to TIS2.
As regards Hypothesis 3 (The RFS latent variables [PVs] show statistically significant [p < 0.05] indirect negative effects and statistically insignificant direct effects (i.e. full mediation) on TIS via mediators JSS and ACS), the analysis produced the results discussed below.
When we regressed all RFS variables, ACS and JSS on TIS2 (Table 5, row 5), the estimated regression paths showed that latent variables CO (β5 = −0.17, p < 0.05) and W (β6 = −0.12, p < 0.05) had statistically significant direct negative effects on TIS2 as a unique contributor to the regression model. The remaining variables, namely, C (β1 = 0.04, p > 0.05), J (β2 = 0.10, p > 0.05), T (β3 = 0.11, p > 0.05) and S (β4 = −0.08, p > 0.05), had statistically insignificant direct effects on TIS2. The low regression coefficients obtained on TIS2 were statistically insignificant compared to the statistically significant and higher regression coefficients obtained on TIS1 (compare rows 3 and 5 in Table 5). The results suggested a possible full mediating role of JSS and ACS in the relationship between C, J, CO, W and the Crt variable TIS2. The combined direct and indirect effects explained 65% of the variance on TIS2 (R2 = 0.65).
The indirect effects of C on TIS2 via ACS (β1 = −0.17, p < 0.05) and of C on TIS2 via JSS (β1 = −0.09, p < 0.05) were both statistically significant (Table 5, rows 6 and 7). Given that both indirect effects were statistically significant, we concluded that both JSS and ACS contributed significantly in fully mediating the path between C and TIS2 (β1 = −0.04, p > 0.05; Table 5, row 5).
The indirect effect of J on TIS2 via JSS (β2 = −0.12, p < 0.05) was statistically significant, but not the indirect effect of J on TIS2 via ACS (β2 = −0.05, p > 0.05; Table 5, rows 6 and 7). Given that only the former indirect effect was statistically significant, JSS most likely fully mediated the path between J and TIS2 (β2 = 0.10, p > 0.05; Table 5, row 5).
The indirect effect of W on TIS2 via JSS (β6 = 0.06, p < 0.05) was statistically significant, but not the indirect effect of W on TIS2 via ACS (β6 = 0.01, p > 0.05; Table 5, rows 6 and 7). Given that only the former indirect effect was statistically significant, only JSS contributed significantly as a partial mediator to the indirect effect of W on TIS2 (β6 = 0.06, p < 0.05).
In summary, the results on indirect effects suggested that both the mediators JSS and ACS contributed to fully mediating the direct effect of C on TIS, and that only JSS fully mediated the direct effect of J on TIS, thus, partially supporting Hypothesis 3. Variables CO, T and S appeared not to support Hypothesis 3 because there were no statistically significant indirect or direct effects on TIS via the mediators JSS and ACS. The direct effect of W on TIS was partially mediated by JSS. Career opportunities showed only a direct effect on TIS, with no evidence of JSS or ACS mediating the effect.
Discussion
Outline of the results
This study addressed the need to analyse multiple latent ER variables and attitudinal antecedents of voluntary turnover in tandem. We used extended SEM techniques to gain a more comprehensive understanding of the simultaneous effect of multiple ER factors on attitudinal antecedents of voluntary turnover. As Lee et al. (2018) emphasise, advanced statistical analyses are important in contributing to a full and deep understanding of the multifaceted nature of ER factors and in enhancing ER management in organisations.
This study indicated the RFS measurement model as being a plausible representation of theory and having the required breadth and depth to ensure sufficient construct validity and enhanced measurement precision. Contrary to conventional models of CFA, the restricted EwC model allowed for trivial but statistically significant cross loadings. According to Hsu et al. (2014), ignoring cross loadings above 0.13 in conventional CFA models can lead to substantial overestimation of pattern coefficients, bloated covariances between factors and biases in path coefficient estimates in SEM models.
Our study confirmed previous research findings (Ansari, 2011; Das & Baruah, 2013; Kashyap & Rangnekar, 2014; Mehmood, Ahmad, Irum, & Ashfaq, 2016) that compensation, job characteristics, work–life balance and CO are, as ER factors, unique predictors of attitudinal antecedents of voluntary turnover. These ER factors explained a large proportion of variance on each of the attitudinal antecedents of voluntary turnover (from 44% to 61%). Remarkably similar to the findings of the meta-analysis studies reported earlier, the path coefficients between compensation, job characteristics, work–life balance and the attitudinal antecedents of voluntary turnover were of a medium to large effect size, confirming the generalisability of our findings. The study supported the notion that ER factors might be distal determinants of turnover intention (R2 = 0.44) because their effects appeared to be mediated by job satisfaction (R2 = 0.61) and affective commitment (R2 = 0.68) as proximal attitudinal determinants of turnover intention (Duarte et al., 2015; Tett & Meyer, 1993). The combined effect of ER factors, affective commitment and job satisfaction on turnover intention was large (R2 = 0.65), affirming the importance of these variables for ER management.
Similar to other relevant studies (e.g. Luna-Arocas & Camps, 2007; Sager, Griffeth, & Hom, 1998; Samad & Yusuf, 2012), our study found that affective commitment partially mediated the relationship between job satisfaction and turnover intention. Affective commitment had the most significant direct relationship (β = −0.56) with employee turnover intention, followed by job satisfaction (β = −0.27). The size of the reported relationships can likely be attributed to affective commitment relating to the organisation as a whole (a broad concept with a long-term perspective), which in turn partially depends on satisfaction with the job itself (a narrow concept with a short-term perspective) (Farrell & Rusbult, 1981; Kooij, Jansen, Dikkers, & De Lange, 2010). We found that compensation directly related to affective commitment (Meyer & Allen, 1997) and that job satisfaction only partially mediated the relationship between compensation and turnover intention. Thus, the finding emphasises the cardinal importance of compensation in ER management – it was the only ER factor that directly impacted on all the attitudinal antecedents of voluntary turnover (Moncarz et al., 2009; Singh, 2019; Tracey, 2014).
Interestingly, job satisfaction fully mediated the direct path from job characteristics and work–life balance to turnover intention, respectively, suggesting that job satisfaction made a unique contribution to turnover intention and served as a precursor to affective commitment in this context. Moreover, the broader concept of affective commitment may only be influenced indirectly by job characteristics and work–life balance, depending on the job and the associated level of job satisfaction (Farrell & Rusbult, 1981). Hirschfeld (2000) and Lee (2019) have found a direct link between job-related intrinsic motivators (e.g. job characteristics; variety, autonomy, task significance, skill utilisation and self-growth) and the level of job satisfaction, which in turn leads directly to both affective commitment and turnover intention. Similarly, work–life balance directly impacts on intrinsic motivation by allowing for a degree of choice over when, where and how much they work, resulting in increased employee autonomy and job satisfaction, which in turn leads directly to both affective commitment and turnover intention (Mas-Machuca, Berbegal-Mirabent, & Alegre, 2016). This finding is supported by Butts et al. (2013) who show that work–life balance has a more significant direct relationship with job satisfaction than with affective commitment. This finding emphasises the importance of a job-satisfaction-conducive work environment in managing turnover intention directly over the short term and in impacting on affective commitment to the organisation indirectly over the long term. Lee et al. (2018, p. 89) call job satisfaction the ‘cardinal job attitude and prime antecedent to employee turnover’.
This study indicated that training and development, supervisor support and CO did not, as hypothesised, relate substantively to affective commitment and job satisfaction. Therefore, no mediating effects involving these variables were evident. This result is not consistent with previous findings (Gani et al., 2020; Ibidunni et al., 2016; Joāo & Coetzee, 2012; Kraimer et al., 2011; Lee, 2019; Van Dyk & Coetzee, 2012), but it can probably be ascribed to previous studies addressing only one retention factor or a subset of factors (Al-Emadi et al., 2015; De Vos & Meganck, 2009) and not controlling for the effect of multiple ER factors. A statistically significant relationship between two sets of variables may be the product of confounding variables (e.g. compensation and job characteristics), leading to incorrect conclusions about the nature of the relationship.
Our study showed that ER factors might be differently related to attitudinal antecedents of voluntary turnover. Gardner, Wright and Moynihan (2011) made a similar conclusion in their study of voluntary turnover and multiple HR practices, and their multilevel analyses across corporate business units provided valuable insights. They showed that three bundles of HRM practices (skilling, motivation and empowerment) affected voluntary turnover differently and that affective commitment did not mediate the effect of skill-enhancing practices (e.g. training and development) on voluntary turnover. In contrast, affective commitment partially mediated motivational and empowerment-enhancing practices (e.g. compensation, job characteristics and work–life balance). They found that motivational and empowerment-enhancing HRM bundles of practice elicited conventional psychological processes that explained an entire range of outcomes and that skills-related HRM bundles of practice (e.g. training and development) elicited many market processes that might lead to different and even inconsistent outcomes. For example, training and development could improve a person’s employability and marketability within and outside an organisation (Dechawatanapaisal, 2018).
Contrary to the previous findings (Gani et al., 2020; Joāo & Coetzee, 2012; Kraimer et al., 2011; Van Dyk & Coetzee, 2012), our study showed that CO had a unique and direct effect on turnover intention but that affective commitment and job satisfaction did not affect it. We argue that CO may represent a large variety of psychological processes and meanings for different individuals (e.g. relating to career stages, family needs, identity, employment opportunities, market-skill demands, spousal careers) and that employee attitudes (e.g. affective commitment) may be affected differentially (Lee, 2019; Lee et al., 2018).
Interestingly, after controlling for the effect of all the variables in the model, supervisor support did not show unique or incremental effects on any of the attitudinal antecedents of voluntary turnover. This finding contradicts previous findings (Gani et al., 2020; Ibidunni et al., 2016; Lu, Zhao, & While, 2019; Tett & Meyer, 1993; Van Dyk & Coetzee, 2012), showing that psychological processes and the meaning of supervisor support may differ for individuals, depending on the employment context and the impact of supervisor support on the motivational and empowerment ER bundles. For instance, Chaudhuri, Arora and Roy (2020, p. 11) state that ‘supervisor support was considered to be a relevant factor that helped with work–life balance’. However, further research is needed to clarify the findings on supervisor support and ER.
To conclude, we found that by studying multiple ER factors in tandem and controlling for all covariances between the ER factors in an expanded SEM model gave new insights into the uniqueness of each ER factor in predicting the attitudinal antecedents of voluntary turnover. Our findings suggest that ER factors have a differential effect on the attitudinal antecedents of voluntary turnover, which can partly be ascribed to differences in the underlying characteristics of HRM bundles of practice as described by Gardner et al. (2011). Employee retention management could be enhanced by a deep understanding of the differential effects of each ER factor on attitudinal antecedents of voluntary turnover, and gaining such an understanding requires advanced SEM skills to thoroughly test increasingly complicated path models using latent variables (Hom et al., 2017; Lee et al., 2018).
Scientific contribution and practical implications
Our research makes a substantial contribution to existing theory by bringing about a better understanding of the multifaceted interrelations between critical ER factors, job satisfaction, affective commitment and turnover intention through the use of extended SEM techniques. A unique finding we made was that affective commitment and job satisfaction were important mediators that differentially affected the relationship between ER factors and employee turnover intention. The responsibility for the differential effects of ER factors on the attitudinal antecedents of voluntary turnover can probably be attributed to motivational and empowerment-enhancing HRM bundles of practice (i.e. compensation, job characteristics, work–life balance, CO and supervisor support) and skill-related HRM bundles of practice (i.e. training and development). This study has shown that the ER factors of compensation, job characteristics, work–life balance and CO (in the given order of importance) are unique determinants of the attitudinal antecedents of voluntary turnover. Career opportunities, an empowerment-enhancing ER factor, directly affect only the turnover intention, suggesting that individual differences and market forces may affect motivational attitudes (e.g. affective commitment) differently. The fact that training and development and supervisor support were found not to be unique determinants of attitudinal antecedents of voluntary turnover could be the result of employment contexts, individual differences and market processes. To effectively manage ER, management must understand the relative embeddedness of a range of ER factors and prioritise motivational and empowerment-enhancing bundles of practice (e.g. compensation, job characteristics, work–life balance and CO) to impact on attitudinal antecedents of voluntary turnover.
The expanded SEM models tested in this study allowed for increased rigour and accuracy of method in simultaneously controlling for all the effects in the model and in determining the uniqueness and relative contribution (i.e. embeddedness) of each variable. The said models provided a new perspective on the relationship between ER factors and attitudinal antecedents of voluntary turnover. The use of the EwC measurement model was a novel approach to applying ESEM to smaller samples whilst retaining the breadth and depth of the measured constructs. Applying PVs in SEM studies with smaller samples is a new approach that counters a variety of potential estimation problems in SEM models and simplifies a complex model without loss of accuracy.
Future studies should also follow a ‘methodological–substantive synergy’ approach and focus on the complex relationship between ER factors and attitudinal proxy variables of voluntary turnover in more contexts and relate it to actual voluntary turnover.
Limitations and recommendations
A limitation of the study is the use of convenience sampling techniques, the cross-sectional nature of the data and the probability that some of the findings may be specific to the sample and the population. Testing causal relations is a common limitation when using cross-sectional data, and making deductions (with caution) must be based on an influential theory and extensive prior empirical evidence, as was performed in this study. Future ER studies could utilise longitudinal or experimental and control group study designs, which may produce more solid findings on causal or mediating effects on employee turnover. However, each of the study designs referred to has its own strengths, limitations and practical implications, which should be considered carefully before conducting a study (see Kline, 2015, for a detailed discussion).
Conclusion
To conclude, we heeded the call of De Vos and Meganck (2009), Dechawatanapaisal (2018), Lee et al. (2018) and others to demonstrate the value of studying multiple ER factors in tandem to better understand the relative embeddedness of a range of ER practices in retaining employees. In addition, we indicated that the use of extended SEM techniques readily met the need for studying ER factors using more comprehensive latent multivariate approaches and for providing new insights into the relationships between ER factors and attitudinal antecedents of voluntary turnover. The findings of this study have important practical implications for effectively managing ER through prioritising and implementing more effective HR bundles of practice.
Acknowledgements
The authors would like to acknowledge Ricole Verreyne who collected the data as a student researcher as part of her Master’s mini-dissertation.
Competing interests
The authors have declared that no competing interests exist.
Author’s contributions
P.S. conceptualised the study, conducted the statistical analyses and drafted the methods section, analyses section and results section of the article. C.O. aided in the conceptualisation of the article and assisted in drafting the literature review. She further aided in refining the article.
Funding information
This research received no specific grant from any funding agency in the public, commercial or not-for-profit sectors.
Data availability statement
Data sharing is not applicable to this article as no new data were created or analysed in this study.
Disclaimer
The views and opinions expressed in this article are those of the authors and do not necessarily reflect the official policy or position of any affiliated agency of the authors.
References
Agho, A.O., Price, J.L., & Mueller, C.W. (1992). Discriminant validity of measures of job satisfaction, positive affectivity and negative affectivity. Journal of Occupational and Organizational Psychology, 65(3), 185–195. https://doi.org/10.1111/j.2044-8325.1992.tb00496.x
Aladwan, K., Bhanugopan, R., & D’Netto, B. (2015). The effects of human resource management practices on employees’ organisational commitment. International Journal of Organizational Analysis, 23(3), 472–492. https://doi.org/10.1108/IJOA-11-2014-0822
Al-Emadi, A.A.Q., Schwabenland, C., & Wei, Q. (2015). The vital role of employee retention in human resource management: A literature review. IUP Journal of Organizational Behavior, 14(3), 7–33. https://doi.org/10.1108/hrmid-11-2019-0272
Allen, N.J., & Meyer, J.P. (1990). The measurement and antecedents of affective, continuance and normative commitment to the organization. Journal of Occupational Psychology, 63(1), 1–18. https://doi.org/10.1111/j.2044-8325.1990.tb00506.x
Anderson, J.C.J., & Gerbing, D.D.W. (1988). Structural equation modeling in practice: A review and recommended two-step approach. Psychological Bulletin, 103(3), 411–423. https://doi.org/10.1037/0033-2909.103.3.411
Ansari, N.G. (2011). Employee perception of HRM practices: Impact on commitment to the organization. South Asian Journal of Management, 18(3), 122–149.
Asparouhov, T., & Muthén, B. (2009). Exploratory structural equation modeling. Structural Equation Modeling, 16(3), 397–438. https://doi.org/10.1080/10705510903008204
Asparouhov, T., & Muthén, B. (2010). Plausible values for latent variables using Mplus. Mplus Technical Report. Retrieved from http://www.statmodel.com/download/Plausible.pdf
Bernhard, F., & O’Driscoll, M.P. (2011). Psychological ownership in small family-owned businesses: Leadership style and nonfamily-employees’ work attitudes and behaviors. Group & Organization Management, 36(3), 345–384. https://doi.org/10.1177/1059601111402684
Bontis, N., Richards, D., & Serenko, A. (2011). Improving service delivery: Investigating the role of information sharing, job characteristics, and employee satisfaction. Learning Organization, 18(3), 239–250. https://doi.org/10.1108/09696471111123289
Butts, M.M., Casper, W.J., & Yang, T.S. (2013). How important are work-family support policies? A meta-analytic investigation of their effects on employee outcomes. Journal of Applied Psychology, 98(1), 1–25. https://doi.org/10.1037/a0030389
Chan, S.H.J., & Ao, C.T.D. (2019). The mediating effects of job satisfaction and organizational commitment on turnover intention, in the relationships between pay satisfaction and work-family conflict of casino employees. Journal of Quality Assurance in Hospitality and Tourism, 20(2), 206–229. https://doi.org/10.1080/1528008X.2018.1512937
Chaudhuri, S., Arora, R., & Roy, P. (2020). Work-life balance policies and organisational outcomes: A review of literature from the Indian context. Industrial and Commercial Training, 52(3), 155–170. https://doi.org/10.1108/ICT-01-2019-0003
Chew, J., & Chan, C.C.A. (2008). Human resource practices, organizational commitment and intention to stay. International Journal of Manpower, 29(6), 503–522. https://doi.org/10.1108/01437720810904194
Cohen, J. (1987). Statistical power of analysis for the behavioral sciences. Hillsdale, NJ: Lawrence Erlbaum Associates.
Ćulibrk, J., Delić, M., Mitrović, S., & Ćulibrk, D. (2018). Job satisfaction, organizational commitment and job involvement: The mediating role of job involvement. Frontiers in Psychology, 9(132), 1–12. https://doi.org/10.3389/fpsyg.2018.00132
Das, B.L., & Baruah, M. (2013). Employee retention: A review of literature. IOSR Journal of Business and Management, 14(2), 8–16. https://doi.org/10.9790/487X-1420816
Dechawatanapaisal, D. (2018). Examining the relationships between HR practices, organizational job embeddedness, job satisfaction, and quit intention: Evidence from Thai accountants. Asia-Pacific Journal of Business Administration, 10(3), 130–148. https://doi.org/10.1108/APJBA-11-2017-0114
Deery, M. (2008). Talent management, work-life balance and retention strategies. International Journal of Contemporary Hospitality Management, 20(7), 792–806. https://doi.org/10.1108/09596110810897619
Devlieger, I., & Rosseel, Y. (2017). Factor score path analysis: An alternative for SEM? Methodology, 13(Suppl 1), 31–38. https://doi.org/10.1027/1614-2241/a000130
De Vos, A., & Meganck, A. (2009). What HR managers do versus what employees value. Personnel Review, 38(1), 45–60. https://doi.org/10.1108/00483480910920705
Döckel, A. (2003). The effect of retention factors on organisational commitment: An investigation of high technology employees. Master’s dissertation. University of Pretoria. Retrieved from https://repository.up.ac.za/bitstream/handle/2263/27597/dissertation.pdf?sequence=1
Döckel, A., Basson, J.S., & Coetzee, M. (2006). The effect of retention factors on organisational commitment: An investigation of high technology employees. SA Journal of Human Resource Management, 2(4), 20–28. https://doi.org/10.4102/sajhrm.v4i2.91
Duarte, A.P., Gomes, D.R., & Neves, J.G. (2015). Satisfaction with human resource management practices and turnover intention in a five-star hotel: The mediating role of perceived organizational support. Dos Algarves: A Multidisciplinary E-Journal, 25(1), 103–123. Retrieved from http://www.dosalgarves.com/revistas/N25/6rev25.pdf
Farkas, A.J., & Tetrick, L.E. (1989). A three-wave longitudinal analysis of the causal ordering of satisfaction and commitment on turnover decisions. Journal of Applied Psychology, 74(6), 855–868. https://doi.org/10.1037/0021-9010.74.6.855
Farrell, D., & Rusbult, C.E. (1981). Exchange variables as predictors of job satisfaction, job commitment, and turnover: The impact of rewards, costs, alternatives, and investments. Organizational Behavior and Human Performance, 28(1), 78–95. https://doi.org/10.1016/0030-5073(81)90016-7
Gani, K., Potgieter, I., & Coetzee, M. (2020). Dispositions of agency as explanatory mechanisms of employees’ satisfaction with retention practices. Journal of Psychology in Africa, 30(2), 143–150. https://doi.org/10.1080/14330237.2020.1744278
Gardner, T.M., Wright, P.M., & Moynihan, L.M. (2011). The impact of motivation, empowerment, and skill-enhancing practices on aggregate voluntary turnover: The mediating effect of collective affective commitment. Personnel Psychology, 64(2), 315–350. https://doi.org/10.1111/j.1744-6570.2011.01212.x
Ghosh, P., Satyawadi, R., Prasad Joshi, J., & Shadman, M. (2013). Who stays with you? Factors predicting employees’ intention to stay. International Journal of Organizational Analysis, 21(3), 288–312. https://doi.org/10.1108/IJOA-Sep-2011-0511
Good, L.K., & Fairhurst, A.E. (1999). Met expectations during role transitions of retail executive trainees. International Journal of Retail & Distribution Management, 27(9), 350–361. https://doi.org/10.1108/09590559910292799
Gorsuch, R.L. (1983). Factor analysis (2nd edn.). Hillsdale, NJ: L. Erlbaum.
Grewal, R., Cote, J.A., & Baumgartner, H. (2004). Multicollinearity and measurement error in structural equation models: Implications for theory testing. Marketing Science, 23(4), 519–529. https://doi.org/10.1287/mksc.1040.0070
Guchait, P., & Cho, S. (2010). The impact of human resource management practices on intention to leave of employees in the service industry in India: The mediating role of organizational commitment. International Journal of Human Resource Management, 21(8), 1228–1247. https://doi.org/10.1080/09585192.2010.483845
Guzeller, C.O., & Celiker, N. (2019). Examining the relationship between organizational commitment and turnover intention via a meta-analysis. International Journal of Culture, Tourism, and Hospitality Research, 14(1), 102–120. https://doi.org/10.1108/IJCTHR-05-2019-0094
Hackman, J.R., & Oldham, G.R. (1976). Motivation through the design of work: Test of a theory. Organizational Behavior and Human Performance, 16(2), 250–279. https://doi.org/10.1016/0030-5073(76)90016-7
Hellgren, J., Sjöberg, A., & Sverke, M. (1997). Intention to quit: Effects of job satisfaction and job perceptions. In F. Avallone, J. Arnold, & K. de Witte (Eds.), Feelings work in Europe (pp. 415–423). Milano: Guerini.
Heneman, H.G., & Schwab, D.P. (1985). Pay satisfaction: Its multidimensional nature and measurement. International Journal of Psychology, 20(1), 129–141. https://doi.org/10.1080/00207598508247727
Hirschfeld, R.R. (2000). Does revising the instrinsic and extrinsic subscales of the Minnesota Satisfaction Questionnaire Short Form make a difference? Educational and Psychological Measurement, 60(2), 255–270. https://doi.org/10.1177/0013164403258442
Hom, P., Lee, T.W., Shaw, J.D., & Hausknecht, J.P. (2017). One hundred years of employee turnover theory and research. Journal of Applied Psychology, 102(3), 530–545. https://doi.org/10.1037/apl0000103
Hom, P.W., Mitchell, T.R., Lee, T.W., & Griffeth, R.W. (2012). Reviewing employee turnover: Focusing on proximal withdrawal states and an expanded criterion. Psychological Bulletin, 138(5), 831–858. https://doi.org/10.1037/a0027983
Homans, G. (1961). Social behavior: Its elementary forms. London: Routledge & Kegan Paul.
Howard, J.L., Gagné, M., Morin, A.J.S., & Forest, J. (2018). Using bifactor exploratory structural equation modeling to test for a continuum structure of motivation. Journal of Management, 44(7), 2638–2664. https://doi.org/10.1177/0149206316645653
Hsu, H.Y., Skidmore, S.T., Li, Y., & Thompson, B. (2014). Forced zero cross-loading misspecifications in measurement component of structural equation models: Beware of even ‘small’ misspecifications. Methodology, 10(4), 138–152. https://doi.org/10.1027/1614-2241/a000084
Ibidunni, S., Osibanjo, O., Adeniji, A., Salau, O.P., & Falola, H. (2016). Talent retention and organizational performance: A competitive positioning in Nigerian banking sector. Periodica Polytechnica, Social and Management Sciences, 24(1), 1–13. https://doi.org/10.3311/PPso.7958
Joāo, T.F., & Coetzee, M. (2012). Job retention factors, perceived career mobility and organisational commitment in the South African financial sector. Journal of Psychology in Africa, 22(1), 69–76. https://doi.org/10.1080/14330237.2012.10874523
Joseph, D., Ng, K-Y., Koh, C., & Ang, S. (2007). Turnover of information technology professionals: A narrative review, meta-analytic structural equation modeling, and model development. MIS Quarterly, 31(3), 547–577. https://doi.org/10.1055/s-0029-1225353
Juhdi, N., Pa’wan, F., & Hansaram, R.M.K. (2013). HR practices and turnover intention: The mediating roles of organizational commitment and organizational engagement in a selected region in Malaysia. International Journal of Human Resource Management, 24(15), 3002–3019. https://doi.org/10.1080/09585192.2013.763841
Kashyap, V., & Rangnekar, S. (2014). A structural equation model for measuring the impact of employee retention practices on employee’s turnover intentions: An Indian perspective. South Asian Journal of Human Resources Management, 1(2), 221–247. https://doi.org/10.1177/2322093714549109
Kelley, H.H., & Thibaut, J.W. (1978). Interpersonal relations: A theory of interdependence. New York, NY: Wiley.
Kim, H., & Kao, D. (2014). A meta-analysis of turnover intention predictors among U.S. child welfare workers. Children and Youth Services Review, 47(P3), 214–223. https://doi.org/10.1016/j.childyouth.2014.09.015
Kline, R.B. (2015). The mediation myth. Basic and Applied Social Psychology, 37(4), 202–213. https://doi.org/10.1080/01973533.2015.1049349
Kochanski, J., & Ledford, G. (2001). How to keep me: Retaining technical professionals. Research Technology Management, 44(3), 31–38. https://doi.org/10.1080/08956308.2001.11671427
Kooij, D.T.A.M., Jansen, P.G.W., Dikkers, J.S.E., & De Lange, A.H. (2010). The influence of age on the associations between HR practices and both affective commitment and job satisfaction: A meta-analysis. Journal of Organizational Behavior, 31(8), 1111–1136. https://doi.org/10.1002/job
Kraimer, M.L., Seibert, S.E., Wayne, S.J., & Liden, R.C. (2011). Antecedents and outcomes of organizational support for development: The critical role of career opportunities. Journal of Applied Psychology, 96(3), 485–500. https://doi.org/10.1037/a0021452
Landau, J., & Hammer, T.H. (1986). Clerical employees’ perceptions of interorganizational career opportunities. Academy of Management Journal, 29(2), 385–404. https://doi.org/10.2307/256194
Larkin, I.M., Brantley-Dias, L., & Lokey-Vega, A. (2016). Job satisfaction, organizational commitment and turnover intention of online teachers in the K-12 setting. Online Learning, 20(3), 26–52. https://doi.org/10.24059/olj.v20i3.986
Lee, H-W. (2019). A career stage analysis of the U.S. federal employees’ job satisfaction and turnover intention: A comprehensive overview. Review of Public Personnel Administration, 40(4), 717–742. https://doi.org/10.1177%2F0734371X19856082
Lee, T.W., Hom, P., Eberly, M., & Li, J. (2018). Managing employee retention and turnover with 21st century ideas. Organizational Dynamics, 47(2), 88–98. https://doi.org/10.1016/j.orgdyn.2017.08.004
Lu, H., Zhao, Y., & While, A. (2019). Job satisfaction among hospital nurses: A literature review. International Journal of Nursing Studies, 94, 21–31. https://doi.org/10.1016/j.ijnurstu.2019.01.011
Lüdtke, O., Robitzsch, A., & Trautwein, U. (2018). Integrating covariates into social relations models: A plausible values approach for handling measurement error in perceiver and target effects. Multivariate Behavioral Research, 53(1), 102–124. https://doi.org/10.1080/00273171.2017.1406793
Luna-Arocas, R., & Camps, J. (2007). A model of high performance work practices and turnover intentions. Personnel Review, 37(1), 26–46. https://doi.org/10.1108/00483480810839950
Mai, Y., Zhang, Z., & Wen, Z. (2018). Comparing exploratory structural equation modeling and existing approaches for multiple regression with latent variables. Structural Equation Modeling: A Multidisciplinary Journal, 25(4), 737–749. https://doi.org/10.1080/10705511.2018.1444993
Marsh, H.W., & Hau, K-T. (2007). Applications of latent-variable models in educational psychology: The need for methodological-substantive synergies. Contemporary Educational Psychology, 32(1), 151–170. https://doi.org/10.1016/j.cedpsych.2006.10.008
Marsh, H.W., Hau, K-T., & Wen, Z. (2004). In search of golden rules: Comment on hypothesis-testing approaches to setting cutoff values for fit indexes and dangers in overgeneralizing Hu and Bentler’s (1999) findings. Structural Equation Modeling: A Multidisciplinary Journal, 11(3), 320–341. https://doi.org/10.1207/s15328007sem1103_2
Marsh, H.W., Morin, A.J.S., Parker, P.D., & Kaur, G. (2014). Exploratory structural equation modeling: An integration of the best features of exploratory and confirmatory factor analysis. Annual Review of Clinical Psychology, 10, 85–110. https://doi.org/10.1146/annurev-clinpsy-032813-153700
Marsh, H.W., Muthén, B., Asparouhov, T., Lüdtke, O., Robitzsch, A., Morin, A.J.S., & Trautwein, U. (2009). Exploratory structural equation modeling, integrating CFA and EFA: Application to students’ evaluations of university teaching. Structural Equation Modeling: A Multidisciplinary Journal, 16(3), 439–476. https://doi.org/10.1080/10705510903008220
Mas-Machuca, M., Berbegal-Mirabent, J., & Alegre, I. (2016). Work-life balance and its relationship with organizational pride and job satisfaction. Journal of Managerial Psychology, 31(2), 586–602. https://doi.org/10.1108/JMP-09-2014-0272
Matz, A.K., Woo, Y., & Kim, B. (2014). A meta-analysis of the correlates of turnover intent in criminal justice organizations: Does agency type matter? Journal of Criminal Justice, 42(3), 233–243. https://doi.org/10.1016/j.jcrimjus.2014.02.004
McDonald, R.P. (1999). Test theory: A unified treatment. New York, NY: Routledge.
McKnight, D.H., Phillips, B., & Hardgrave, B.C. (2009). Which reduces IT turnover intention the most: Workplace characteristics or job characteristics? Information and Management, 46(3), 167–174. https://doi.org/10.1016/j.im.2009.01.002
Mehmood, N., Ahmad, U.N.B.U., Irum, S., & Ashfaq, M. (2016). Job satisfaction, affective commitment, and turnover intentions among front desk staff: Evidence from Pakistan. International Review of Management and Marketing, 6(4S), 305–309. Retrieved from https://www.econjournals.com/index.php/irmm/article/view/2503/pdf
Meyer, J.P., & Allen, N.J. (1991). A three-component conceptualization of organizational commitment. Human Resource Management Review, 1(1), 61–89. https://doi.org/10.1016/1053-4822(91)90011-Z
Meyer, J.P., & Allen, N.J. (1997). Commitment in the workplace: Theory, research, and application. London: Sage.
Meyer, J.P., & Herscovitch, L. (2001). Commitment in the workplace: Toward a general model. Human Resource Management Review, 11(3), 299–326. https://doi.org/10.1016/S1053-4822(00)00053-X
Meyer, J.P., Stanley, D.J., Herscovitch, L., & Topolnytsky, L. (2002). Affective, continuance, and normative commitment to the organization: A meta-analysis of antecedents, correlates, and consequences. Journal of Vocational Behavior, 52, 20–52. https://doi.org/10.1006/jvbe.2001.1842
Moncarz, E., Zhao, J., & Kay, C. (2009). An exploratory study of US lodging properties’ organizational practices on employee turnover and retention. International Journal of Contemporary Hospitality Management, 21(4), 437–458. https://doi.org/10.1108/09596110910955695
Morin, A.J.S., Marsh, H.W., & Nagengast, B. (2013). Exploratory structure equation modeling. In G.R. Hancock, & R.O. Mueller (Eds.), Quantitative methods ineducation and the behavioral sciences: Issues, research, and teaching. Structural equation modeling: A second course (2nd edn., pp. 395–436). Charlotte, NC: IAP Information Age Publishing.
Muthén, L.K., & Muthén, B. (2002). How to use a Monte Carlo study to decide on sample size and determine power. Structural Equation Modeling, 9(4), 599–620. https://doi.org/10.1207/S15328007SEM0904_8
Muthén, L.K., & Muthén, B. (2012). Mplus user’s guide (7th edn.). Retrieved from https://www.statmodel.com/download/usersguide/Mplus%20user%20guide%20Ver_7_r3_web.pdf
Narayanan, A., Rajithakumar, S., & Menon, M. (2019). Talent management and employee retention: An integrative research framework. Human Resource Development Review, 18(2), 228–247. https://doi.org/10.1177/1534484318812159
Nohe, C., & Sonntag, K. (2014). Work-family conflict, social support, and turnover intentions: A longitudinal study. Journal of Vocational Behavior, 85(1), 1–12. https://doi.org/10.1016/j.jvb.2014.03.007
Nouri, H., & Parker, R.J. (2020). Turnover in public accounting firms: A literature review. Managerial Auditing Journal, 35(2), 294–321. https://doi.org/10.1108/MAJ-03-2018-1823
Potgieter, I.L., & Snyman, A.M. (2018). Personal factors in talent retention in the South African banking industry. Journal of Psychology in Africa, 28(6), 455–461. https://doi.org/10.1080/14330237.2018.1544393
Price, J.L., & Mueller, C.W. (1981). A causal model for turnover for nurses. Academy of Management Journal, 24(3), 543–565. https://doi.org/10.2307/255574
Ramus, C.A., & Steger, U. (2000). The roles of supervisory support behaviors and environmental policy in employee ‘ecoinitiatives’ at leading-edge European companies. Academy of Management Journal, 43(4), 605–626. https://doi.org/10.2307/1556357
Rathi, N., & Lee, K. (2017). Understanding the role of supervisor support in retaining employees and enhancing their satisfaction with life. Personnel Review, 46(8), 1605–1619. https://doi.org/10.1108/PR-11-2015-0287
Richman, A.L., Civian, J.T., Shannon, L.L., Jeffrey Hill, E., & Brennan, R.T. (2008). The relationship of perceived flexibility, supportive work-life policies, and use of formal flexible arrangements and occasional flexibility to employee engagement and expected retention. Community, Work & Family, 11(2), 183–197. https://doi.org/10.1080/13668800802050350
Rogg, K.L., Schmidt, D.B., Shull, C., & Schmitt, N. (2001). Human resource practices, organizational climate, and customer satisfaction. Journal of Management, 27(4), 431–449. https://doi.org/10.1177/014920630102700403
Rubenstein, A.L., Eberly, M.B., Lee, T., & Mitchell, T.R. (2015). Looking beyond the trees: A meta-analysis and integration of voluntary turnover research. Academy of Management Proceedings, 2015(1), 12779–12779. https://doi.org/10.5465/AMBPP.2015.20
Rubenstein, A.L., Eberly, M.B., Lee, T.W., & Mitchell, T.R. (2017). Surveying the forest: A meta-analysis, moderator investigation, and future-oriented discussion of the antecedents of voluntary employee turnover. Personnel Psychology, 71(1), 23–65. https://doi.org/10.1111/peps.12226
Rubin, D.B. (1987). Multiple imputation for nonresponse in surveys. New York, NY: Wiley.
Saber, D.A. (2014). Frontline registered nurse job satisfaction and predictors over three decades: A meta-analysis from 1980 to 2009. Nursing Outlook, 62(6), 402–414. https://doi.org/10.1016/j.outlook.2014.05.004
Sager, J.K., Griffeth, R.W., & Hom, P.W. (1998). A comparison of structural models representing turnover cognitions. Journal of Vocational Behavior, 53(2), 254–273. https://doi.org/10.1006/jvbe.1997.1617
Samad, S., & Yusuf, S.Y.M. (2012). The role of organizational commitment in mediating the relationship between job satisfaction and turnover intention. European Journal of Social Sciences, 30(1), 125–135. https://doi.org/10.33071/ssricb.42.1.201804.99
Sánchez-Oliva, D., Morin, A.J.S., Teixeira, P.J., Carraça, E.V., Palmeira, A.L., & Silva, M.N. (2017). A bifactor exploratory structural equation modeling representation of the structure of the basic psychological needs at work scale. Journal of Vocational Behavior, 98, 173–187. https://doi.org/10.1016/j.jvb.2016.12.001
Sheraz, F., Batool, S., & Adnan, S. (2019). Employee’s retention and job satisfaction: Mediating role of career development programs. Dialogue, 14(2), 67–78. Retrieved from http://www.qurtuba.edu.pk/thedialogue/The%20Dialogue/14_2/07-saima.pdf
Shockley, K.M., & Singla, N. (2011). Reconsidering work-family interactions and satisfaction: A meta-analysis. Journal of Management, 37(3), 861–886. https://doi.org/10.1177/0149206310394864
Singh, D. (2019). A literature review on employee retention with focus on recent trends. International Journal of Scientific Research in Science, Engineering and Technology, 6(1), 425–431. https://doi.org/10.32628/ijsrst195463
Sjöberg, A., & Sverke, M. (1996). Predicting turnover intention among nurses: The role of work values. In V.V. Baba (Ed.), Work values and behaviour: Research and applications (pp. 213–223). Montreal, Canada: International Society for the Study of Work and Organizational Values.
Smeenk, S.G.A., Eisinga, R.N., Teelken, J.C., & Doorewaard, J.A.C.M. (2006). The effects of HRM practices and antecedents on organizational commitment among university employees. International Journal of Human Resource Management, 17(12), 2035–2054. https://doi.org/10.1080/09585190600965449
Solinger, O., Van Olffen, W., & Roe, R. (2008). Beyond the three-component model of organizational commitment. Journal of Applied Psychology, 93(1), 70–83. https://doi.org/10.1037/0021-9010.93.1.70
Subramony, M. (2009). A meta-analytic investigation of the relationship between HRM bundles and firm performance. Human Resource Management, 48(5), 745–768. https://doi.org/10.1002/hrm.20315
Paré, G., Tremblay, M., & Lalonde, P. (2001). The role of organizational commitmentand citizenship behaviors in understanding relations between human resources practices and turnover intentions of IT personnel. Retrieved from www.cirano.qc.ca/files/publications/2001s-24.pdf.
Tett, R.P., & Meyer, J.P. (1993). Job satisfaction, organizational commitment, turnover intention, and turnover: Path analyses based on meta-analytic findings. Personnel Psychology, 46(2), 259–293. https://doi.org/10.1111/j.1744-6570.1993.tb00874.x
Thurstone, L.L. (1947). Multiple factor analysis. Chicago, IL: University of Chicago.
Tracey, B.J. (2014). A review of human resources management research. International Journal of Contemporary Hospitality Management, 26(5), 679–705. https://doi.org/10.1108/IJCHM-02-2014-0056
Valaei, N., & Rezaei, S. (2016). Job satisfaction and organizational commitment: An empirical investigation among ICT-SMEs. Asia Pacific Journal of Marketing and Logistics, 39, 1–55. https://doi.org/10.1108/MRR-09-2015-0216
Van Dyk, J., & Coetzee, M. (2012). Retention factors in relation to organisational commitment in medical and information technology services. SA Journal of Human Resource Management, 10(2), 1–11. https://doi.org/10.4102/sajhrm.v10i2.433
|